Speakers
Distinguished Guest Speakers
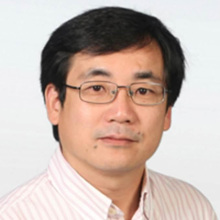 |
Hai Yang
Chair Professor
Department of Civil and Environmental Engineering
Hong Kong University of Science and Technology
|
Title: Competitive ride-sourcing markets with a third-party integrator
Abstract: With the rapid development of shared mobility, third-party integrators have emerged recently to integrate competitive platforms that offer on-demand ride services in ride-sourcing markets. With platform integration, a passenger can request a ride through the integrator and can be matched with an idle driver affiliated to any of the integrated platforms, whichever is first or closer. As a result, passengers can access a higher level of supply, generating a thicker market with shorter average matching and pickup times. More importantly, platform integration maintains a competitive environment in which the participating platforms still set their prices, wages, and other operating strategies independently. In this paper, we propose mathematical models to characterize the Nash equilibrium of the ride-sourcing market with multiple competing platforms and compare market outcomes with and without platform-integration. We find that platform-integration can increase total realized demand and social welfare but may not necessarily generate a greater profit when vehicle supply is sufficiently large or/and market is too fragmented.
|
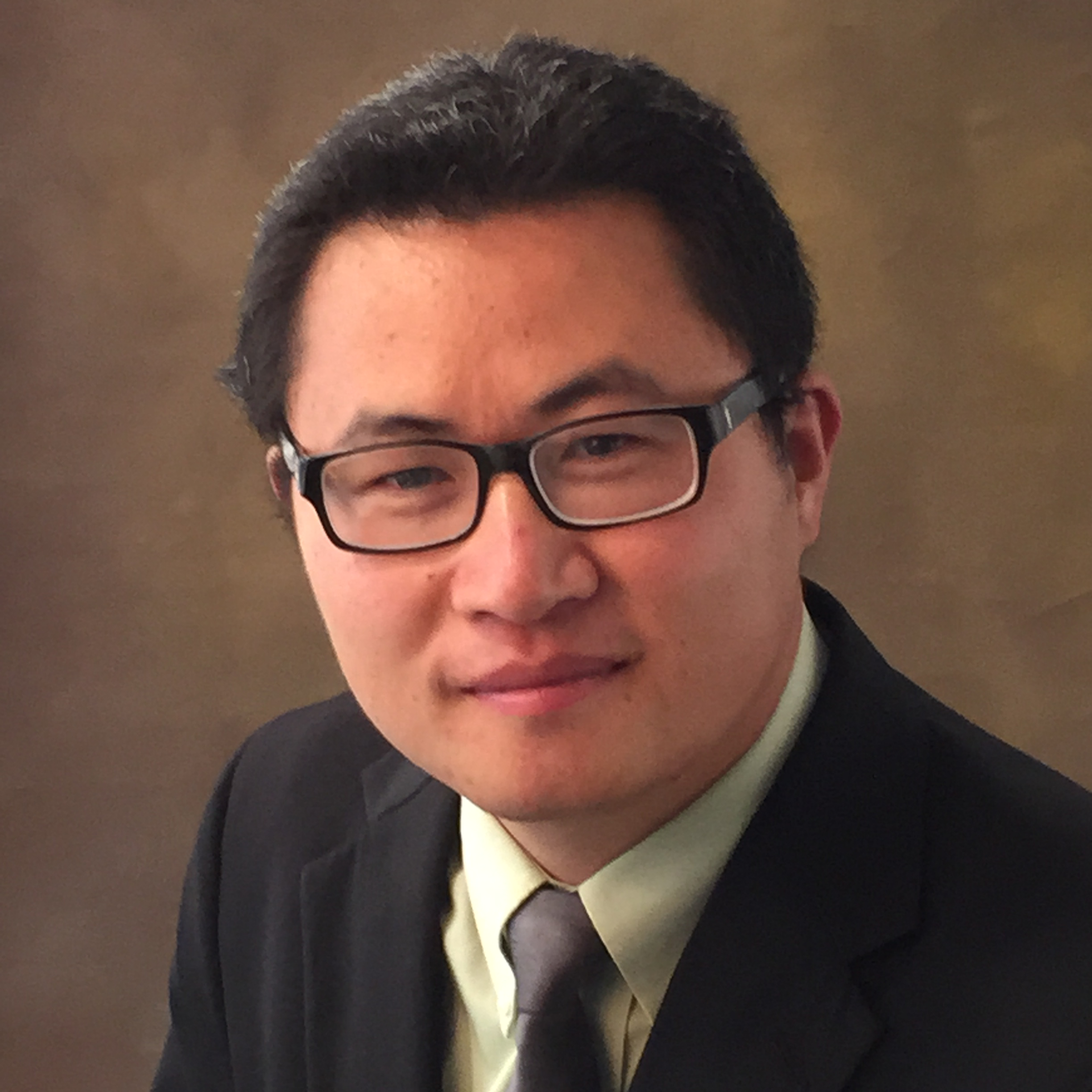 |
Yanfeng Ouyang
George Krambles Endowed Professor
Civil and Environmental Engineering
1209 Newmark Civil Engineering Laboratory
University of Illinois at Urbana-Champaign
|
Title: Design of demand-responsive mobility services: From flexible transit to ridesharing
Abstract: The talk presents a few efforts on designing demand-responsive mobility service systems that provide door-to-door transportation in cities. These systems include combinations of traditional transit, nonshared taxis, paratransit services (such as dial-a-ride), and newer forms of ridesharing (shared taxis or carpooling) used by crowd-sourced companies like DiDi and Uber. Operational similarities and differences of these systems will be discussed, along with quantitative comparison of their resource requirements (e.g., the required operating fleet size) and passengers’ expected level of service (e.g., average door-to-door travel time). We also show how our models can help mobility service providers and government agencies systematically explore operating, pricing, and regulatory strategies to enhance sustainability of urban transportation.
|
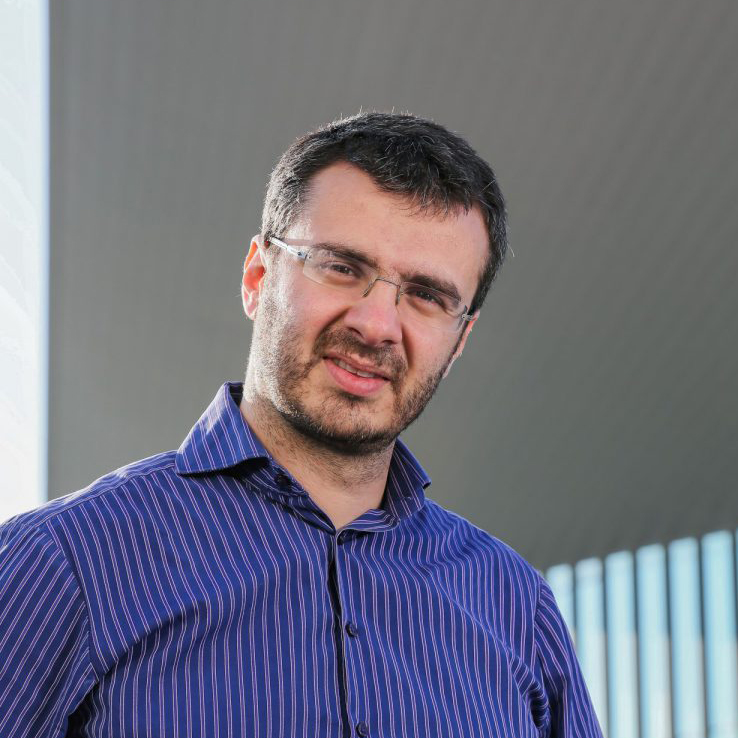 |
Nikolas Geroliminis
Associate Professor
Urban Transport Systems Laboratory
École polytechnique fédérale de Lausanne
|
Title: On the inefficiency of ride-sourcing services towards urban congestion
Abstract: The advent of shared-economy and smartphones made on-demand transportation services possible, which created additional opportunities, but also more complexity to urban mobility. Companies that offer these services are called Transportation Network Companies (TNCs) due to their internet-based nature. Although ride-sourcing is the most notorious service TNCs provide, little is known about to what degree its operations can interfere in traffic conditions, while replacing other transportation modes, or when a large number of idle vehicles is cruising for passengers. We experimentally analyze the efficiency of TNCs using taxi trip data from a Chinese megacity and an agent-based simulation with a trip-based MFD model for determining the speed. We investigate the effect of expanding fleet sizes for TNCs, passengers’ inclination towards sharing rides, and strategies to alleviate urban congestion. We observe that, although a larger fleet size reduces waiting time, it also intensifies congestion, which, in turn, prolongs the total travel time. Such congestion effect is so significant that it is nearly insensitive to passengers’ willingness to share and flexible supply. Finally, parking management strategies can prevent idle vehicles from cruising without assigned passengers, mitigating the negative impacts of ride-sourcing over congestion, and improving the service quality. |
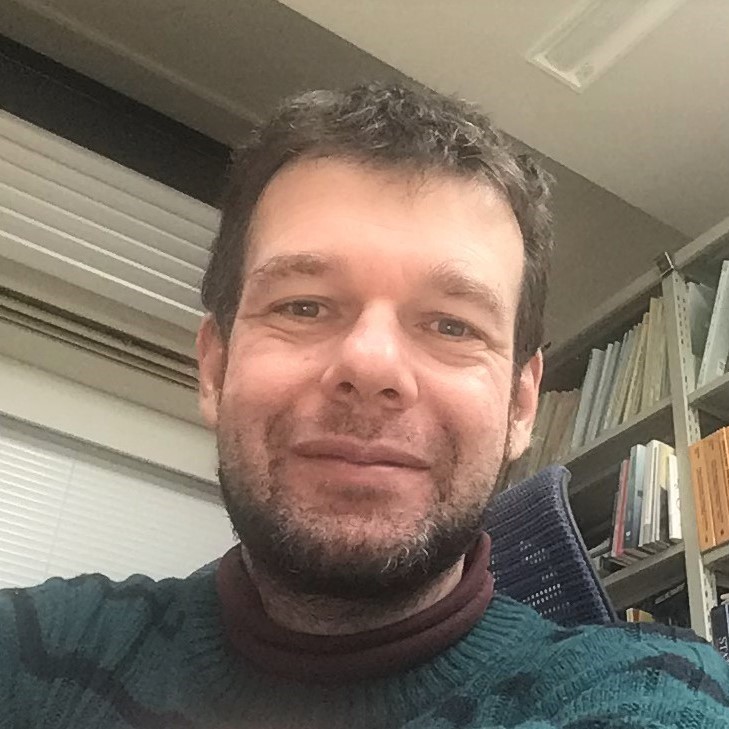 |
Jan-Dirk Schmoecker
Associate Professor
Department of Urban Management
Kyoto University
|
Title: Estimating tourism trip patterns in Kyoto with map data and popularity ratings
Abstract: In this presentation I discuss ongoing research activities in our research group to estimate travel behavioural patterns of tourists in Kyoto. We utilise GPS traces as well as Wi-Fi sensor data to observe routes of visitors within the city. To explain these we establish behavioural models in which we consider the attractiveness of touristic areas in the city based on information obtainable from OpenStreetmap and Google information about "points of interest" in the area. We show that "user ratings" as well as the type of POIs can explain roue choice and the duration with which people stay at certain locations to some degree. Heuristic approaches of estimating trips as a large numerical problem versus using a recursive logit approach are discussed. The presentation further includes comments on the impact of COVID19 on travel patterns in the city. |
Invited Guest Speakers
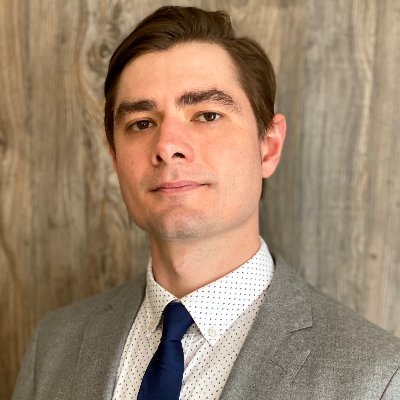 |
Lewis James Lehe
Assistant Professor
Civil and Environmental Engineering
University of Illinois at Urbana-Champaign
|
Title: Hyperdemand: how shared rides make demand curves bend backwards
Abstract: Static traffic models, in the tradition of Walters (1961), typically feature a ‘‘demand curve’’ giving the vehicle flow demanded for each unit travel time (inverse speed). Traditionally, the demand curve declines because people want to drive more as travel times fall. In this talk, I show vehicle flow demanded can, instead, plausibly rise with unit travel time (a phenomenon called ‘‘hyperdemand’’), if congestion induces some people to switch from high-to low-occupancy modes. Consequently, multiple equilibria can arise even in light congestion, and even temporary policies can have permanent effects by moving traffic to a different equilibrium path. |
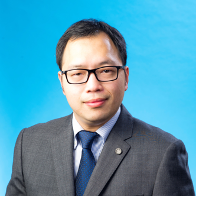 |
Andy Chow
Assistant Professor
Department of Architecture and Civil Engineering
City University of Hong Kong
|
Title: Urban transit operations with use of reinforcement learning
Abstract: In this talk, we will present some recent work on use of reinforcement learning (RL) techniques for managing urban transit operations with circulation of limited rolling stock in a stochastic environment. The problem considered herein is modelled as a Markov decision process. With consideration of the underlying 'curses of dimensionality', we develop a RL-based computational framework which simplifies the evaluation and searching process for potential optimal control policies in real time by parameterizing the state and decision spaces. The proposed approach is tested with various real-world case studies. Experiment results illustrate the advantages of the proposed method over a range of situations with unexpected uncertainties including the pandemic scenarios. This study innovates urban transit operations with state-of-the-art computer science and dynamic optimization techniques. |
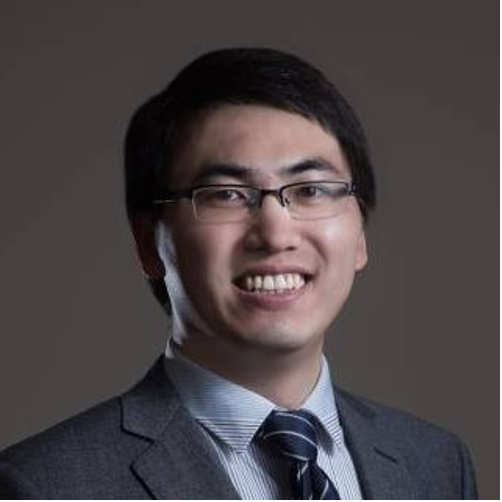 |
Xiqun (Michael) Chen
"Hundred Talents Program" Professor
College of Civil Engineering and Architecture
Zhejiang University
|
Title: Regulating on-demand ride-sourcing services: Two-period dynamic game and agent-based simulation
Abstract: Regulating on-demand ride-hailing services (e.g., Uber and DiDi) requires a balance of multiple competing objectives: encouraging innovative business models (e.g., DiDi), sustaining traditional industries (e.g., taxi), creating new jobs, and reducing traffic congestion. By analyzing a two-period dynamic game that involves these stakeholders, we find that, without government intervention, the on-demand ride service platform can drive the traditional taxi industry out of the market under certain conditions. Relative to no regulations and a complete ban policy, a carefully designed regulatory policy can strike a better balance of multiple competing objectives. Data-driven agent-based modeling and simulation for large-scale transportation networks are implemented to investigate how regulatory policies impact the ride-sourcing market, which goes beyond existing approaches by employing data-driven multi-objective deep learning to train ride-sourcing drivers' offline/online behavior. The modeling and simulation system has the potential to help decision-makers and ride-sourcing platforms to assess regulatory policies and operations management strategies in the era of shared mobility.
|
For a replay of the workshop record, please follow the link with a password of "3ZHPC^e7": Replay.
|